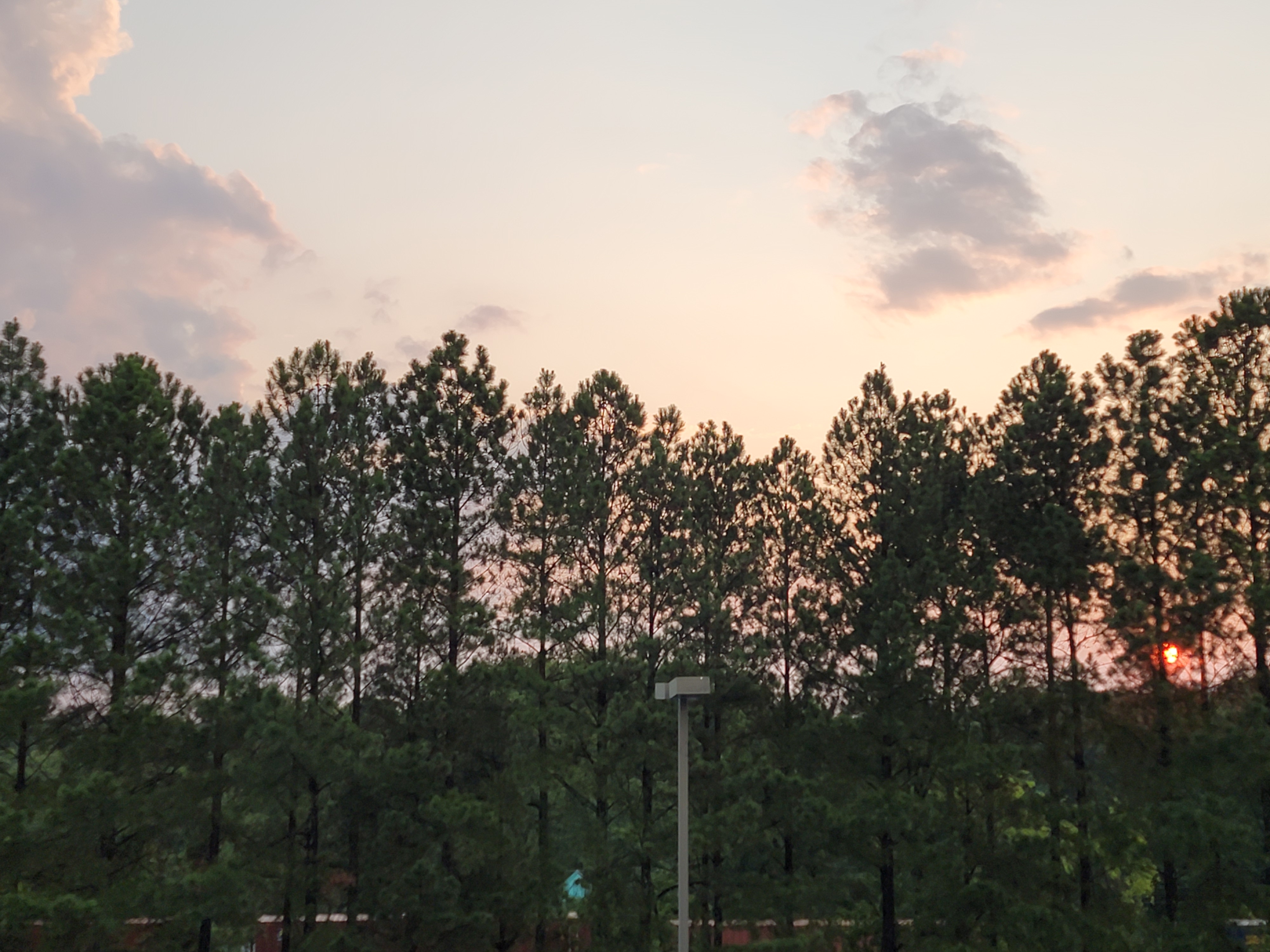
graduate student researcher
Researching galactic coevolution as a NASA FINESST Fellow in Dr. John Wise's Computational Cosmology group. I'm currently focused on using machine learning techniques to emulate star formation and feedback in large volume hydrodynamic cosmological simulations, with the goal of accelerating simulation runtime while retaining large-scale physics fidelity. My interests are not confined to cosmology; more broadly, I would love to apply my computational expertise on large, real datasets and use any scientific training I've learned towards solving modern problems, either in astrophysics or any related field, such as climate modeling. The things that keep me coming back to the office are the amazing mentees, peers, and mentors I get to work with and learn from, the cool resources I have the privilege of abusing, and the constant need to buy food and toys for the four legged furballs that occupy my home rent-free. When I'm not working, you might find me reading or writing about fantasy worlds (that we have yet to discover, some magic-believers might say...), making music, teaching dance, or generally overcommitting myself to things!
Typically what my work week would consist of
Professional appointments & research projects
Trained production team and new engineers on videotaping & editing software, usage of professional-quality cameras, and handling of tricaster technology. Chief engineer on Wake Up Rutgers, a student-run morning news show that occurred twice weekly. Maintained fiber network and servers for Rutgers television and internet services. Communicated administratively between engineering, production, and programming teams.
Worked with Dr. Rachel Somerville and Dr. Ena Choi. Analyzed hydrodynamic simulation outputs of supermassive galaxies between redshifts 0 - 2, and determined trends in mass-to-light ratios of these galaxies. The goal of the whole project was to understand the effect of feedback from the active galactic nucleus (AGN) on star formation rates. Completed capstone honors thesis on this project and earned high honors.
Worked with Dr. Stephen Zepf and Dr. Mark Peacock. Using the Virgo Galaxy Cluster (VGC), conducted photometry as well as SDSS data munging and cleaning to obtain a catalog of ~400 globular clusters that existed in both SDSS and Hubble Space Telescope (HST) databases and were observed with the 'same' filter. Using these data, determined a gradient existed between the two telescopes' filters and determined this gradient value for HST's F475W and SDSS's F850LP filters when observing the VGC. Presented a poster for the project at the Princeton CUWiP 2017 and won a poster competition.
Tutored high school and undergrad students on various physics and math subjects as well as python coding. Had 23 students totally consistently helped students raise their grades by at least 10%.
Trained an artificial neural network (ANN) to use only galactic properties as input to determine a spectral energy distribution for simulated galaxies, replacing radiative transfer calculations. Current iteration of the ANN is applicable for IllustrisTNG galaxies and the scripts to use it have been released on github. Paper published in Monthly Notices of the Royal Astronomical Society in 2023.
Worked to create mock observations of a simulated high-redshift galaxy both with and without a central AGN to determine observational differences between a galaxy with a quiescent AGN and a galaxy without an AGN. Completed a group project exploring the contribution of central black holes to the galactic spectrum of high-redshift galaxies observed by JWST. Thesis work is creating a star formation and feedback emulator using machine learning techniques in order to accelerate large-scale hydrodynamic cosmological simulations while retaining physics fidelity.
For any professional inquiries, please email me or refer to socials below.